Focus
Using digital data to affect learning experiencesPrincipal Metaphors
Different proponents of Learning Analytics align with different theories of learning, and so metaphors of learning and learners can vary dramatically from one publication to the next. That said, there are persistent calls for optimization and efficiency, as well as broad use of the Attainment Metaphor – which might be taken as indications that “learning” is somewhat undertheorized within the discourse.Originated
2010sSynopsis
Learning Analytics is most often understood in terms of collecting and analyzing data on learners and their situations, in order to improve their learning. (To this end, some versions of Learn Analytics align strongly with Design-Based Learning and Design Thinking.) Learning Analytics is principally focused on E-Learning and other engagements that have pronounced digital components, which enable tracking of learner choices, time on task, patterns of error, interactions among different regions of the brain, and so on. Related discourses and relevant constructs include:- Academic Analytics (1990s) – the analysis of data furnished by universities and other institutions, aimed at improving student success, increased accountability to stakeholders, and gauging of institutional quality
- Data Fishing – the disreputable practice of subjecting a data set to a barrage of statistical tests and then reporting only those that present significant results
- Data Masking (De-Identified Data; Masked Data) – the stripping or encrypting of information, usually to conceal identities of individuals, schools, or jurisdictions. Most often, Data Masking is undertaken so that information on demographics or performance can be made available to researchers, consultants, or other interested parties.
- Datafication – any and every effort to digitize aspects of social and natural worlds
- Disaggregated Data – information that has been processed to reveal trends and patterns that might otherwise be indiscernible within large data sets – on, for example, gender- or race-based differences in educational opportunities and achievements. The processing involves collecting data (from multiple sources, across multiple variables), aggregating data (i.e., creating summaries), and disaggregating data (i.e., parsing into meaningful components).
- Educational Data Mining (2010s) – variously defined, but most often understood to encompass both Learning Analytics and Academic Analytics. Educational Data Mining encompasses both the techniques and the field of study designed to tap the increasingly vast repositories of data derived from (or related to) people’s educational activities, with the intention of affecting learning and/or the experience of learning in some positive way.
- Epistemic Frame Theory (D.W. Shaffer, A.F. Wise; 2010s) – a version of Learning Analytics that is specifically aligned with Community of Practice
- Student Information System – any digital system designed to track, store, and relay relevant schooling information (e.g., grades, attendance) on students
-
Student-Level Data (Individual-Level Data; Individual Student-Level Data; Record-Level Data; Record-Level Student Data; Student Unit-Level Data; Student-Unit Record Data; Unit-Record Data) – information collected by educational agents and agencies on individual students, including (but not limited to) personal data, achievement scores, course completions, disciplinary problems, medical history, and psychological testing. Such data are used for purposes that include tailoring educational programs, allocating funding for schools, ranking jurisdictions, and informing educational policy.
- Digital Rights (World Summit on the Information of Society [WSIS], 2000s) – the extent to which one can access, utilize, and contribute to digital resources (inc. media, devices, networks, etc.) while maintaining conventional rights (e.g., to privacy, to self-expression, to ownership of personal information)
Commentary
Proponents of Learning Analytics tend to be consistent in aligning the discourse with data science and design, and somewhat varied with regard to commitments around learning and teaching. Indeed, as intimated above, the phenomenon of learning tends to be undertheorized within the discourse. Consequently, it would be an overstatement to suggest that those engaged in Learning Analytics share either a vision of education or a theory of learning.Authors and/or Prominent Influences
George Siemens; Dragan GaševićStatus as a Theory of Learning
Learning Analytics does not aim to offer any new insight into the dynamics and complexities of learning.Status as a Theory of Teaching
While there are varying opinions of what constitutes learning among proponents of Learning Analytics, advocates do tend to agree that statistical analysis of large amounts of data can be used to improve whatever learning is defined to be. That is, the discourse is principally concerned with influencing learning.Status as a Scientific Theory
We have color-coded Learning Analytics as amber because of the varied and often-uncritical manners in which the notion of “learning” is deployed. That said, it is a robust domain of inquiry with a rapidly growing empirical base, and it appears to be evolving toward a rigorous scientific domain.Subdiscourses:
- Academic Analytics
- Data Fishing
- Data Masking (De-Identified Data; Masked Data)
- Datafication
- Digital Rights
- Disaggregated Data
- Educational Data Mining
- Epistemic Frame Theory
- Student Information System
- Student-Level Data (Individual-Level Data; Individual Student-Level Data; Record-Level Data; Record-Level Student Data; Student Unit-Level Data; Student-Unit Record Data; Unit-Record Data)
Map Location
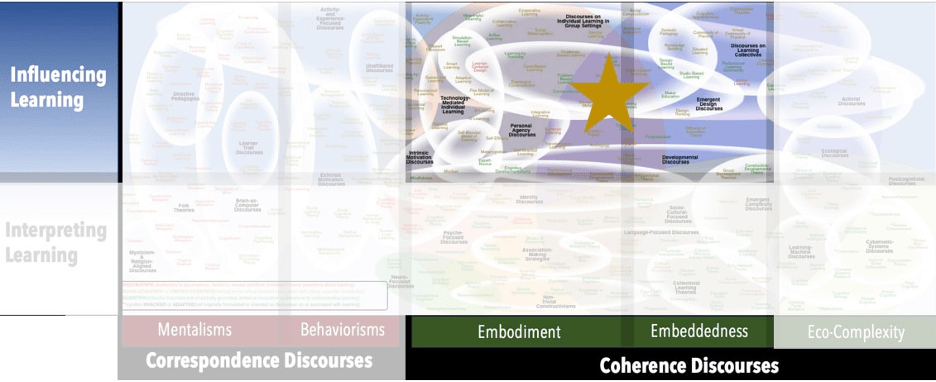
Please cite this article as:
Davis, B., & Francis, K. (2023). “Learning Analytics” in Discourses on Learning in Education. https://learningdiscourses.com.
⇦ Back to Map
⇦ Back to List