AKA
Complex Adaptive Systems
Complexity
Complexity Theory/Thinking/Science
Dynamical Systems Theory
General Systems Theory
Generative Science
Nonlinear Dynamics
Self-Organization Theory
Systems
Systems Research
Systems Science
Systems Thinking/Theory
Focus
Agents forming a unified whole in their interactions, relationships, or dependenciesPrincipal Metaphors
- Knowledge is … range of possible activity
- Knowing is … doing, being
- Learner is … a complex system
- Learning is … adapting
- Teaching is … influencing, occasioning
Originated
1950sSynopsis
As might be inferred from the list of alternative titles at the top of this page, we have collected a range of interconnected field and theories under the umbrella of Complex Systems Research. A complex system comprises a group of agents that forms a unified whole in their interactions, relationships, or dependencies. The emergent behavior of that grander unity is transcendent; it exceeds the possibilities of any agents on their own, and it cannot be predicted on the basis of the rules governing those agents. In this regard, critical notions include:- Attractor State (1980s) – a stable mode of systemic activity. Systems that have achieved Attractor States tend to remain stable, even when modestly disturbed.
- Adaptive Capacity – the extent to which a system is able to learn – that is, to modify itself – in order to take advantage of emergent possibility, to overcome unexpected challenge, or to otherwise respond to novel circumstances
- Antifragility (Nassim Taleb, 2010s) – a property of complex systems whereby they thrive due to stresses, errors, failures, instabilities, and/or assaults. Antifragility is distinguished from Resilience (the ability to cope with and recover from crisis situations; under Grit).
- Self-Organization (Self-Organized Criticality) (Per Bak, Chao Tang, Kurt Wiesenfeld, 1980s) – a feature of a complex system that appears as relatively stable macro-behavior, even while micro-activity (i.e., interactivities of its subsystems) can vary dramatically. Self-Organized Criticality occurs without centralized control.
b
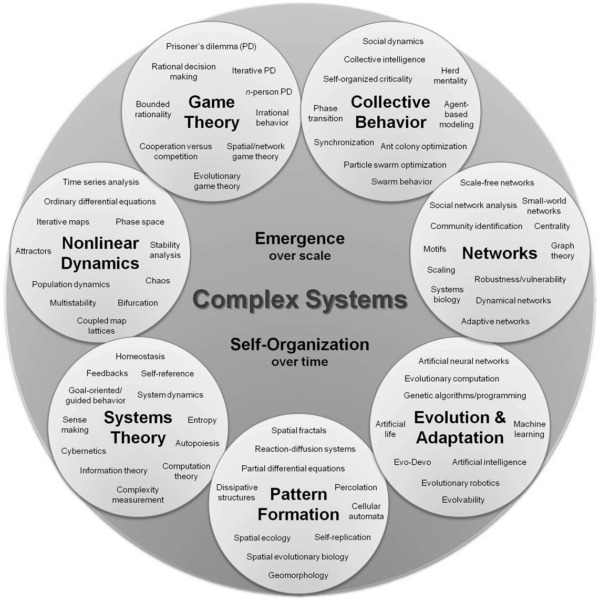
b
Beyond those listed in the above graphic, associated constructs and discourses include:- Agent-Based Modeling (Individual-Based Modeling) – a computational approach for studying the behavior of a system by simulating the actions of the agents that it comprises
- Anthropo-Complexity (Dave Snowden, 2020s) – the domains of complexity that have been influenced or shaped by human Modes of Reasoning, which are argued to be characteristically distinct from both biological systems (e.g., those associated with insects or jungle ecosystems) and agent-based computational models
- Assembly Theory (Leroy Cronin, 2020s) – a perspective on the emergence of complexity in nature, as governed by the laws of evolution. Assembly Theory proposes indices that suggest how complex the assembly of a form (e.g., molecule) might be, which in term is offered as an indicator of the probability that form is associated with a living entity. Assembly Theory was developed initially to detect evidence of life in off-world settings, and it is now being used to assess lab-based efforts to evolve new life forms.
- Catastrophe Theory (René Thom, 1960s) – a mathematical theory that attends to sudden, significant changes that are triggered by very small changes. (In matters of learning, it has been applied to the relationship between performance and, for example, matters of anxiety or arousal.) Popularizations of the notion include:
- Tipping Point (Malcolm Gladwell, 2000s) – a threshold point in a complex system at which multiple, very-diverse possibilities present themselves – and at which point an explosive and irreversible systemic transformation may occur. Within discussions of learning, the notion of Tipping Point is often invoked as a challenge to educational structures based on assumptions of smooth, incremental, linear progress.
- Critical Juncture Theory – Defining “critical junctures” as major turning points in the evolution of some form or phenomenon, Critical Junction Theory is used in various Social Sciences (see Sociology) to identify, describe, and explain sociocultural structures and dynamics.
- Chaos Theory (Edward Lorenz, 1960s) – a transdisciplinary field of scientific inquiry that is concerned with identifying and understanding patterns within the apparent randomness that can emerge in dynamical systems as small changes in conditions trigger disproportionate effects. Among many educators, Chaos Theory has been embraced to make sense of such phenomena as learners’ wildly different interpretations of very similar experiences, the hard-to-pin-down nature of the “teachable moment,” and the impossibility of “perfect lessons.” Prominent associated constructs include:
- Butterfly Effect (Edward Lorenz, 1960s) – a quality of some complex systems whereby its state is massively dependent on its “initial conditions.” That is, based on an analogy to the triggering of a storm in one part of the world by the flapping of a butterfly’s wings in another part, the Butterfly Effect asserts that minor changes to its starting conditions can lead to major and unpredictable differences in outcomes in complex systems.
- Fractal (Benoît Mandelbrot, 1980s) – a descriptive term that can be applied to any shape that has the same “bumpiness of detail” across multiple levels of magnification. That is, a Fractal does not appear simpler or more complicated when magnified or reduced. The notion has found some traction in discussions of learning, as it offers an alternative metaphor to those associated with Reductionism – that is, to the assumption that phenomena must get simpler when parsed or magnified. Associated constructs include:
- Scale Invariance (Scale Independence) – the formation/appearance/use of the same structure at different scales (i.e., levels of magnitication).
- Self-Similarity – a type of Scale Invariance in which an object resembles itself at different levels of magnification. (A familiar example is the way a tree branch can resemble the entire tree.)
- Network Theory (various, 1990s) – Narrowly defined, Network Theory is the mathematical study of relationships of the unities in a system, typically resulting in the generation of image that is useful for extrapolating general properties of the system (e.g., How well can it learn?). More broadly, Network Theory offers insights into different types and dynamics of networks, including the realization that all complex systems have a decentralized network structure. That point is argued to be vital in discussions of learning and well-being, based on the assertion that learning systems are necessarily complex systems. This point is perhaps more comprehensible by comparing network structures, four basic types of which have been identified – all of which are represented in formal education in one way or another, and only one of which is associated with the phenomenon of learning:
- Centralized Network – a network structure with a principal hub through which all relationships (e.g., flow of information, channeling of resources) are mediated. This network structure has the advantage of efficient distribution and communication. However, its disadvantages include that it is only as robust and only as flexible as the central hub. (See simplified image, below.)
- Distributed Network – a network structure with tight and extensive local connectivity, but no largescale systemic connectivity, affording a netlike structure that is robust by in which distribution and communication are very inefficient. Phenomena with this structure are highly resistant to change. (See simplified image, below.)
- Decentralized Network – a network structure that might be construed as having many centers – that is, a network in which many nodes are, in a sense, the centers of their own networks. This network structure combines efficient communication with a robust structure, enabling considerable flexibility and high adaptability. Phenomena with decentralized network structures include languages, social networks, the Internet, and the brain. Decentralized Networks are the “fingerprints” of complex learning systems. (See simplified image, below.) Associated constructs include:
- Centrality – a numeration-based strategy to compare and rank the significance of nodes in a Decentralized Network – for example, to identify highly influential people in a social network, the key hubs in the Internet, or superspreaders of a disease
- Dynamic Networks (Adaptive Networks) – the study of robust, self-organizing networks. The domain blends research into system dynamics from Complex Systems Research with emerging insights from Network Theory.
- Multi-Agent System (Multi-Agent Simulation) – a computational system comprising multiple, relatively autonomous agents in a Decentralized Network. Typically, a Multi-Agent System is designed to solve a problem or perform a search that is beyond the capacity of any single-agent system.
- Scale-Free Network – a network in which the connectivity of the nodes follows a Power Law Distribution (see below). That is, Scale-Free Network has many nodes with few links and few nodes with many links.
- Small-World Network – a type of network in which any given node is likely to be linked to neighboring nodes but much less likely to be linked to distant nodes, all in a manner that makes it possible to get from one node to any other node in a small number of links
- Fragmented Network – a network structure that lacks meta-connectivity – that is, technically, not really a network at all. With regard to complex co-activity, this type of structure can have at least one use/advantage: it can afford isolated explosions of diversity within smaller clusters that might, at some point, come together into a grander network. (See simplified image, below.)
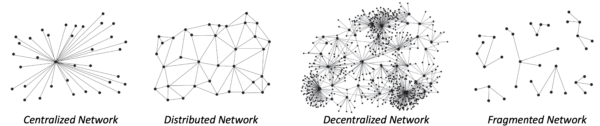
- Power Law Distribution – a mathematical model used to describe those phenomena that have large numbers of small items/members/events and small number of large items/members events, thus indicating that small occurrences are common and larger occurrences are rare. Examples include wealth distribution, earthquakes, learning events, wars, Internet hubs, social trends, life-forms, and, articulations. Mathematically, one quantity varies as a power of another. Types of Power Law Distributions include:
- Pareto Distribution (80/20 Rule) – a type of probability distribution (i.e., theoretical) that describes phenomena in which a small number of occurrences or individuals account for a disproportionately large number of the total effect – often articulated as about 80% of the effects coming from 20% of the causes
- Zipf’s Law – an empirical law (i.e., based on observation) stating that when a set of measured values is organized in order from most frequent to least frequent, the most common value appears twice as often as the second most common value, three times as often as the third, and so on
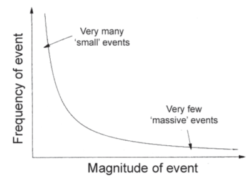
- System Archetypes (Peter Senge, 1990s) – types system dynamics in which Positive Feedback and/or Negative Feedback (see Cybernetics) give rise to common or generic patterns of activity. Among the dozens of System Archetypes that have been described, examples that we’ve encountered in the educational literature include:
- Boiled Frog Syndrome – a System Archetype reflecting the observation that gradual change often goes unnoticed until it’s too late. That is, incremental degradation of conditions can pass unnoticed until the system reaches a crisis point.
- Compensating Feedback (Policy Resistance) – the tendency of some systems to push back against and/or nullify change (i.e., restore previous conditions) as its already-established dynamics assert themselves
- Eroding Goals (Drifting Goals) – a System Archetype in which diminished performances trigger diminished expectations in self-amplifying cycles
- Escalation – a System Archetype in which agents perceive themselves in competition and thus respond to one another in ways that systematically amplify differences and aggressions
- Fixes That Fail – a System Archetype in which a specific solution (“fix”) for a problem has a positive effect in the short-term, but negative effects in the long-term
- Rule Beating – a System Archetype that points to problems that can arise with agents exploit loopholes in rules (i.e., “game the system”) rather than addressing underlying problems
- Seeking the Wrong Goal – a System Archetype highlighting how misaligned metrics can lead to dysfunctional behavior and system degradation
- Shifting the Burden – a System Archetype in which a problem’s symptoms (rather than its underlying causes) are addressed, typically leading to a reliance on whatever means are used to manage those symptoms
- Success to Successful – a System Archetype in which differential resources (e.g., pedagogical support, emotional attention, or physical assets) are provided to agents who show early success, thus amplifying differences between those agents and others
- Tragedy of the Commons (William Forster Lloyd, 1830s) – a System Archetype in which individuals acting in their own self-interest exhaust resources and compromise the common good
- Systemics (Mario Bunge, 1970s) – a general term, initially coined in reference to non-reductive research perspectives. Currently, Systemics is most often used an a synonym for Complex Systems Research.
- Systems Biology – an interdisciplinary domain that embraces a holistic approach in the study of the complex dynamics within biological systems. Associated constructs include:
- Holobiont – a synergetic collective composed of interacting organisms from different species
- Superorganism (Supraorganism) – a synergetic collective composed of interacting organisms of the same species
- System Dynamics – a domain of study, organized around mathematical modeling, that is focused on better understanding the dynamics of complex systems
- Systems Philosophy – an academic domain focused on bringing principles of Complex Systems Research to bear on philosophy
- World-Systems Theory (World-Systems Analysis; World-Systems Perspective; World System Paradigm) (Immanuel Wallerstein, 2000s) – a systems-based approach to societal evolution and world history
- Awareness-Based Systems Change (Awareness-Based Change) (Otto Scharmer, 2010s) – a consciousness-based, collaboration-intensive, and transformation-focused practice concerned with prompting systemic change through examining and shifting the deeper structures of social systems
- Dynamicism (Dynamic Cognition; Dynamic Hypothesis) (Tim van Gelder, 1990s) – the proposal that, within regard to currently available computation-based modeling tools, human cognition is best interpreted using differential equations, nonlinear dynamics, and other aspects of Complex Systems Research
- Functional Dynamic Systems (1990s) – a theoretical framework used to understand complex systems and their behaviors over time. Functional Dynamic Systems emphasizes the interactions and relationships between components of a system, rather than focusing solely on individual elements in isolation.
- Relational Systems Thinking (Melanie Goodchild, 2020s) – a blend of Awareness-Based System Change (see above) and Indigenous Epistemologies (see Decolonizing Education) that is concerned with finding ways for these sensibilities to coexist and co-evolve.
- Rhythmic Intelligence (Michel Alhadeff-Jones, 2020s) – individual and/or collective ability to manage transformational processes by attending to and working with/in the systemic rhythms that operate in their lives
- Systems Sensing (Luea Ritter, 2020s) – a deliberate-but-humble attentiveness to the relational structures and interactive dynamics of a given system, oriented toward wise and meaningful efforts to influence that system
- Systems Thinking Iceberg (Karla Pearson, Dennis Meadows, 1990s) – a four-level framework used to analyze complex systems by visualizing them as an iceberg – i.e., emphasizing that much of the structure will not be immediately available to perception (i.e., lies beneath the surface). The levels are: Events (at the tip of the iceberg, visible occurrences that prompt one to react); Patterns/Trends (just below the water’s surface, predictable happenings that provide context for Events and enable one to anticipate); System Structures (well below the water’s surface, the underlying relationships, rules, or processes that drive Patterns and should figure into design); Mental Models (at the base of the iceberg, beliefs, values, or assumptions that shape the System Structure and that one must work to transform to significantly affect the system).
Commentary
Edgar Morin has offered the following distinction among types of Complex Systems Research:- Restricted Complexity (Edgar Morin, 2000s) – an attitude toward complexity aligned with classical (reductionist) science, focused on identifying underlying laws and structures of systems and using mathematical modeling to predict their behaviors
- General Complexity (Edgar Morin, 2000s) – an attitude toward complexity that acknowledges the inherent unpredictability and interconnectedness of systems, emphasizing the limitations of reductionist approaches. Its focus is on emergent properties and dynamic interactions within systems, accepting that not all aspects can be fully understood or predicted.
Authors and/or Prominent Influences
Yaneer Bar-Yam; Albert-Laszlo Barabasi; Gregory Bateson; Ludwig von Bertalanffy; Murray Gell-Mann; John Holland; Stuart Kauffman; Melanie Mitchell; Steven Strogatz; Duncan Watts; Stephen WolframStatus as a Theory of Learning
With regard to the concerns and foci of education, Complex Systems Research might be described as offering a meta-theory of learning – as the graphic below is intended to suggest.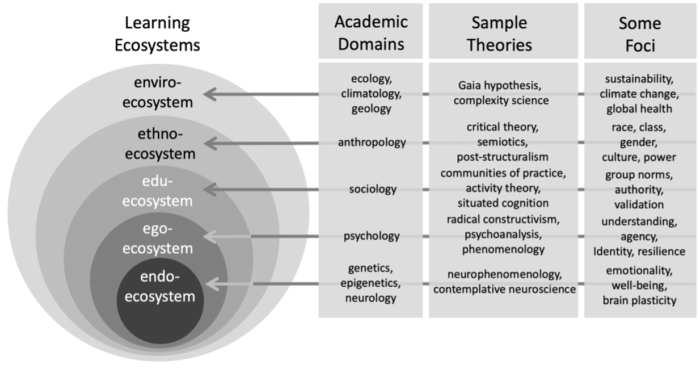
Status as a Theory of Teaching
Complex Systems Research is not a theory of teaching.Status as a Scientific Theory
Complex Systems Research is a robust are of scientific inquiry.Subdiscourses:
- Adaptive Capacity
- Agent-Based Modeling (Individual-Based Modeling)
- Anthropo-Complexity
- Antifragility
- Assembly Theory
- Attractor State
- Awareness-Based Systems Change (Awareness-Based Change)
- Boiled Frog Syndrome
- Butterfly Effect
- Catastrophe Theory
- Centrality
- Centralized Network
- Chaos Theory
- Compensating Feedback (Policy Resistance)
- Critical Juncture Theory
- Decentralized Network
- Distributed Network
- Dynamic Networks (Adaptive Networks)
- Dynamicism (Dynamic Cognition; Dynamic Hypothesis
- Eroding Goals (Drifting Goals)
- Escalation
- Fixes That Fail
- Fractal
- Fragmented Network
- Functional Dynamic Systems
- General Complexity
- Holobiont
- Multi-Agent System (Multi-Agent Simulation)
- Network Theory
- Pareto Distribution (80/20 Rule)
- Power Law Distribution
- Relational Systems Thinking
- Restricted Complexity
- Rhythmic Intelligence
- Rule Beating
- Scale Invariance (Scale Independence)
- Scale-Free Network
- Seeking the Wrong Goal
- Self-Organization (Self-Organized Criticality)
- Self-Similarity
- Shifting the Burden
- Small-World Network
- Success to Successful
- Superorganism (Supraorganism)
- System Archetypes
- System Dynamics
- Systemics
- Systems Biology
- Systems Philosophy
- Systems Sensing
- Systems Thinking Iceberg
- Tipping Point
- Tragedy of the Commons
- World-Systems Theory (World-Systems Analysis; World-Systems Perspective; World System Paradigm)
- Zipf’s Law
Map Location
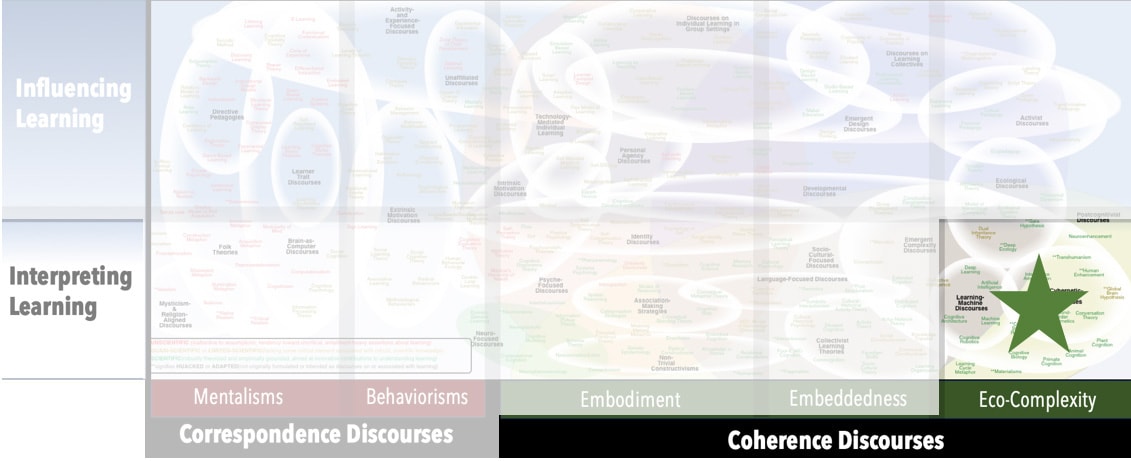
Please cite this article as:
Davis, B., & Francis, K. (2025). “Complex Systems Research” in Discourses on Learning in Education. https://learningdiscourses.com.
⇦ Back to Map
⇦ Back to List